A Breakthrough to Move Cancer Precision Medicine Forward
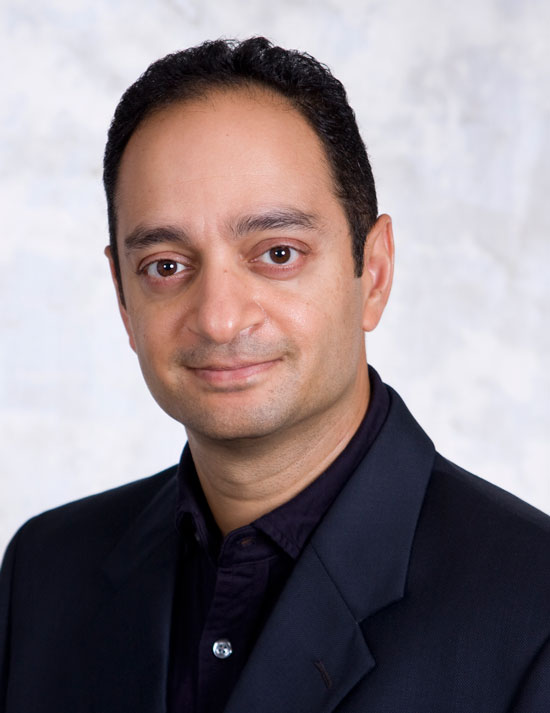
The terms “personalized medicine” and “precision medicine” are often used interchangeably; however, in the world of science, they’re not quite the same. Personalized medicine means developing a unique treatment plan for each patient. Precision medicine means developing treatment plans for groups of patients with similar conditions. Oncologists aspire to personalized medicine, but cancer is like snowflakes — each patient’s disease has its own unique mutations and other traits.
“Physicians would like to identify red-flag mutations and come up with the magical combination that works really well for that one patient,” explains J. Sunil Rao, Ph.D., co-leader of the Cancer Control and Prevention Program at Sylvester Comprehensive Cancer Center, and interim chair and professor of public health sciences at the University of Miami Miller School of Medicine. “It turns out, that’s very challenging because if you look deeply enough at a patient’s tumor, everyone’s cancer is different.”
That’s where precision medicine comes in. Even if clinicians can’t custom-design a treatment plan for a single patient, they can group that person with similar patients to deliver more-targeted therapies. If they can do so accurately, the payoff is that very accurate predictions of patient outcomes can potentially be achieved. That leads to another question: How do physicians ensure that each patient gets slotted to an appropriate group?
Interpreting complex data
It starts with data. Hundreds of thousands of cancer patients have been studied, and information from these previous studies helps determine disease groupings. Theoretically, clinicians can take information about a patient — demographics, family history, genomic and other molecular profiles — and group them accordingly. This is similar to recommendation algorithms companies like Amazon use based on customer purchasing profiles. However, there’s a catch: No patient’s data is complete. There are unknown unknowns.
“In each group, I have things I can explain with a set of variables and things I can’t explain,” said Rao, who is also director of the Division of Biostatistics. “In theory, there are an infinite number of variables, but I can only measure a finite number of them. The leftover information is a type of statistical dark matter.”
When it comes to precision medicine, this dark matter is where the magic lies. Somehow, these unknowns must be accounted for to ensure patients get mapped to appropriate treatment groups for their cancer. Fortunately, Rao, fifth-year biostatistics Ph.D. student Jie Fan, and colleagues at University of California Davis and Oregon Health Sciences University have found a solution. In a recent paper in the Journal of the American Statistical Association, the top journal in the statistical sciences, they describe a method called Classified Mixed Model Prediction (CMMP). This new algorithm can handle the murky data, eventually generating highly accurate individual outcome predictions.
A new approach
CMMP uses a random variable, which calculates the range of possibilities that could make up the statistical dark matter, and then optimally maps this random variable to a group. To prove their point, the researchers applied their algorithm to breast cancer data from the National Cancer Institute’s The Cancer Genome Atlas.
“On real data, it just blows other competitors away,” said Rao. “The errors we make are fewer and far less dramatic. CMMP is demonstrably better. We also characterized the uncertainty of these predictions because you need both pieces of information to properly make decisions. My focus is on cancer, but in theory, it can be used for any disease where you’re trying to make such predictions and want to use the precision medicine framework to do that.”
To translate the methodology to the broader research community, free open-source software will be released soon. Additionally, the methodology will continue to be extended. “There are a number of other interesting precision prediction problems in cancer which this methodology may lend itself to,” said Rao. “We hope to have additional insights soon. This research has been truly enlightening as it’s taught us a lot of new things about how to make accurate predictions in cancer.”
This research was funded in part by NIH grant R01 GM085205A1 and NSF grant SES 1122399.
Original post can be found here.
Leave a Reply